Can AI predict cancer in the future?
- Sam McInerney
- Aug 25, 2024
- 9 min read
Updated: Aug 28, 2024
With the right understanding of genetics, molecular markers, and the impact of other data on cancer development, can personalised medicine translate into personalised predictions?
Or has the hyperbole engine of AI as the saviour of all things reached new lows (or highs depending on your perspective)...
As a flippant statement, it sounds like nonsense... my own knee-jerk response being: 'Of course it can't, that's ridiculous... yet another example of AI being over-egged as the saviour of all things.' But, is it? With enough of 'the right' data, can AI see what we can't see?
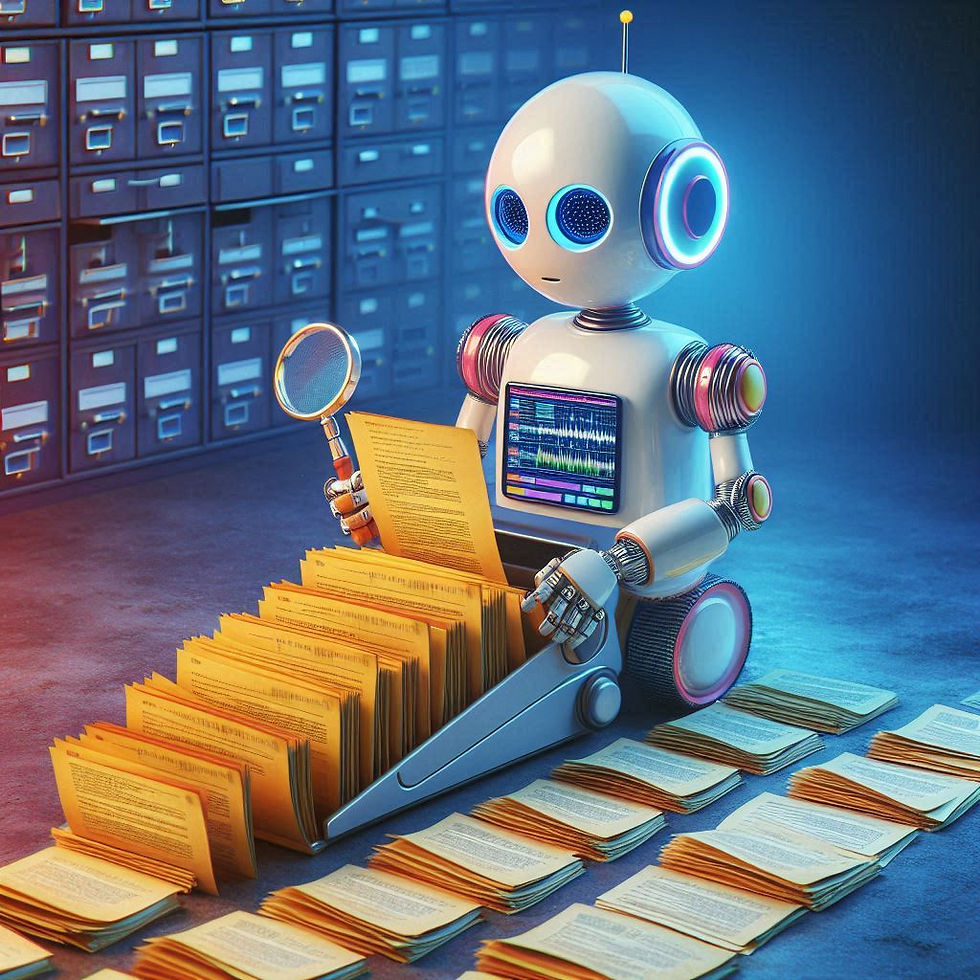
Imagine we put everything useful we know about a patient into a box... The free text we've written about them, the images they've had taken, genetic results, their cancer molecular profile, demographics, social information etc. ... and put the lid on and shake it.
What can all that information tell us about the chances of that specific person developing cancer in the future? The "shaking-the-box" metaphor representing a multimodal AI model cleverly trained and optimised on massive amounts of past patient and cancer information.
It's got to be an idea worth exploring!
With the right understanding of genetics, molecular markers, and the impact of other data on cancer development - can personalised medicine translate into personalised predictions?
Part one of the puzzle is to work out how we determine what is useful and what is noise in the data. We could certainly use AI to help us work that out.
Part two, creating and testing the prediction models.
When you think about it, it's not actually that much of a leap that these data can help us predict future cancers.
After all, we are already answering questions on prognosis and likely outcomes based on trial data. Practically in the clinic, this is where oncologists like myself are manually matching up the patient in front of us, to what is essentially the line of best fit from the trial data we have available. So it's probably not a question of 'if ', but more a question of 'how accurate can we make it?'.
So let's take a look at some of the evidence and tools and out there and see what the lay of the land is.
Predictive tools pre-AI
Prognostic and predictive tools aren't a new idea in medicine, there are plenty of examples out there for various cancer types. A tool used to predict the chance of recurrence and one of the most commonly used in my experience is the 'predict' breast cancer tool: https://breast.predict.cam/
I'd highly recommend having a look and a play with this tool. It gives you a tiny glimpse of what it's like to be a cancer patient. The impossible decision faced by people who experience breast cancer, who need to decide on what therapy's they will try knowing the long list of side effects they can potentially cause.
Given a picture of your own mortality in a set of numbers and probabilities, whereby switching between the tab 'having the chemo' and 'not having the chemo' makes a 5% difference to your chance of being alive in 10 years. Harrowing, to say the least.
The breast predict model was first introduced in 2011, and built on data collected from ~5,200 women.
You use it by entering a few details about a breast cancer patient, it will give you estimates of the overall survival benefit of chemotherapy and hormone therapies. It's had several refits since, and the latest iteration published in Nature reflects the improvement in cancer outcomes and includes radiotherapy. It utilises the complete data from ~35,000 patients, and it's validated on a further ~132,000 patients. [1],[2] Impressive work given it's done manually without AI using classical statistical methods like the Cox proportional hazards (CPH)a .
(As a quick recap - CPH is a statistical technique used in survival analysis to explore the relationship between the survival time of individuals and one or more predictor variables. It is one of the most commonly used models in medical research for time-to-event data, where the "event" is often death, disease progression, or another significant health outcome).
This tool reminds us that we already possess the ability to make predictions without AI. But it's important to remember that for those sorts of numbers, you're looking at a lot of work!
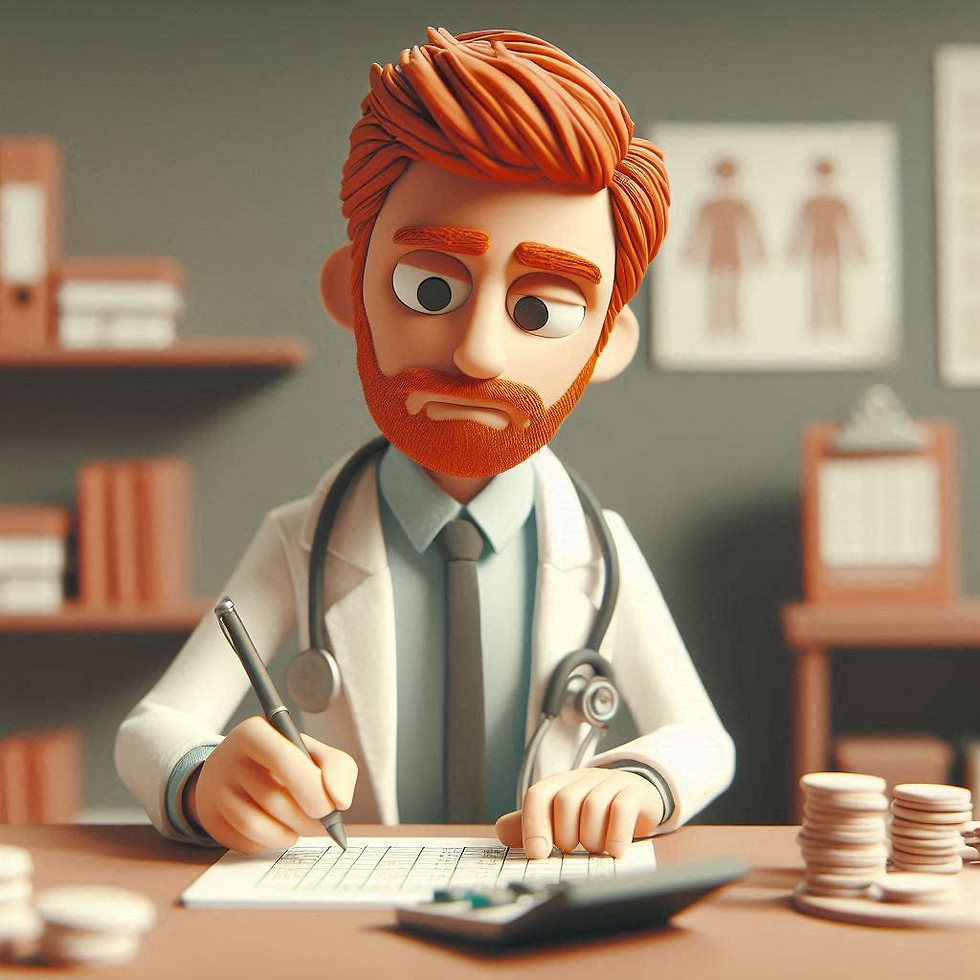
The breast cancer predict tool can be extremely effective in the right situation.
It can help guide conversations about the potential benefits of chemotherapy on survival.
And breast cancer isn't alone, there are a number of non AI based tools out there to help predict the risk of developing cancer or a recurrence.
Whether it's the risk of any cancer based on postcode, symptoms and personal demographics, such as that provided by the QCancer tool, or more specific offerings look at lung cancer risk factors, such as the Liverpool Lung Project (LLP) Model.
So now the age of AI has swept upon us, what more can it offer to the mix? Can it do a better job of reading the future? Let's dig in and find out.
AI-Based Predictive Tools
When I started I had no idea how many people were working on this.
Wow, there are a lot. A few searches in and I found myself overwhelmed by the number of AI tools out there being developed across the cancer spectrum.
Before you jump in and start comparing models, a step back is needed and some thought about where these models sit. What stage are we targeting? Pre-diagnosis, within the diagnostic pathway, or prediction of disease return?
For example, are we looking at individuals in the population who have no known serious problems or cancers and we want to assess lifestyle and known family history risks?
Or perhaps identifying those at higher risk of cancer, based on demographic, social, or lifestyle risks, such as diet, alcohol or smoking?
What about those identified at higher risk for say, lung cancer, at which we may already have a chest X-ray or low-dose CT available for analysis to add to the predictive model?
And finally, those who already have cancer. For which we have complex molecular and genetic data on file, and we are looking to see if they will develop a recurrence after their treatment?
The number of potential targets for this predictive AI is extensive, and the further down the rabbit hole of predictive AI models you go, the more complex the question gets. (And the models for that matter).
Without turning this blog post into an extensive literature review, I decided to focus on a handful of results, but the area that is my main area of excitement is that of deep learning AI technology.
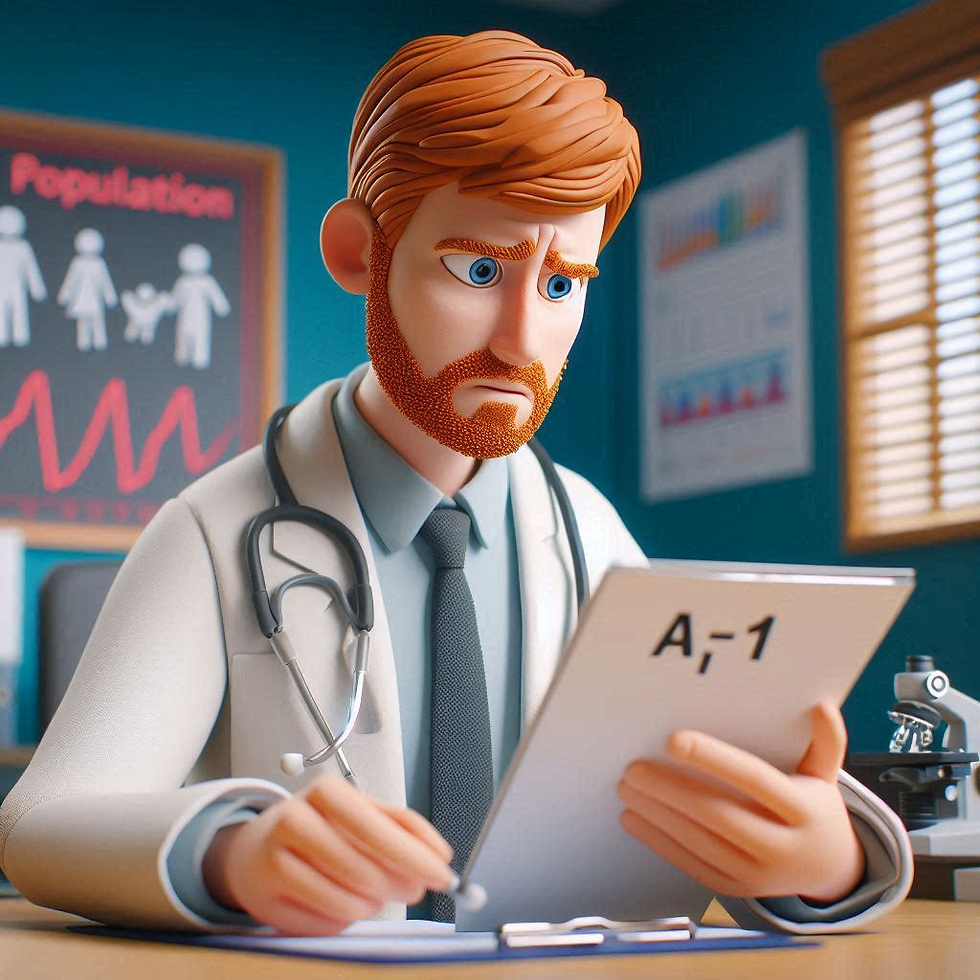
Deep learning
Deep learning represents one of the most advanced capabilities in AI.
With recent advancements in both algorithmic techniques and the hardware enabling parallel processing, deep learning has become especially powerful in areas like cancer detection. Several features make deep learning particularly well-suited for identifying patterns that might be imperceptible to humans:
Complex Pattern Recognition:
Deep learning models, especially deep neural networks, can identify intricate and non-linear patterns in large datasets that traditional machine learning models might overlook.
End-to-End Learning:
These models can learn directly from raw data, reducing the need for manual feature extraction and engineering, which streamlines the development process. This is especially beneficial when working with large-scale, population-level data, where the complexity and volume of information make manual processing impractical.
Scalability:
Deep learning models can handle vast amounts of data and benefit from increased computational power, making them ideal for big data applications.
The Downside:
And it's a really big one. Explainability.
The significant downside of deep learning models is their lack of explainability. These models function as "black boxes," meaning that while they may identify patterns beyond human capability, they often do not provide interpretable reasons for their decisions.
This is a major problem, particularly in healthcare. If a model suggests a high risk of cancer recurrence, it’s crucial to explain why, rather than simply stating, "because the computer says so." Additionally, the issue of accuracy remains a challenge. The phenomenon of "hallucinations" where models generate plausible but incorrect information—is particularly problematic in healthcare. The stakes are too high and, understandably, the industry is too risk-averse to take a chance on hallucinated data.
So what has AI got to offer in the cancer prediction world?
It's probably worth mentioning at this stage that cancer is complicated.
We don't fully understand cancer yet, so the whole story of what matters and what doesn't isn't clear yet, especially when it comes to the fine print.
(And by fine print, all the genes, proteins, the micro-environment, and all the rest of it that make us susceptible to cancer). The latest update on Hanahan et al's infamous Hallmarks of Cancer explains the current landscape of cancer understanding. So this is a challenge in itself for the AI to help solve.
To give a flavour of this complexity, one paper I wrestled with for some time was this one:
Now this paper was pretty challenging to conceptualise without a pretty solid understanding of cancer and the immune system.
To summarise, it's about T cell receptors. End of knowledge. :D hahaha.
Joking aside, they have used deep learning in the form of NLP to identify proteins to predict cancer-related immune status! This will help our understanding of how the immune response behaves against cancer, and in time will give us a great idea of how each individual will respond to potential cancers.
So you can see, that on top of simple parameters like sex, smoking history and age, we've got a lot to play with. And who knows where this might get us in the relatively near future.
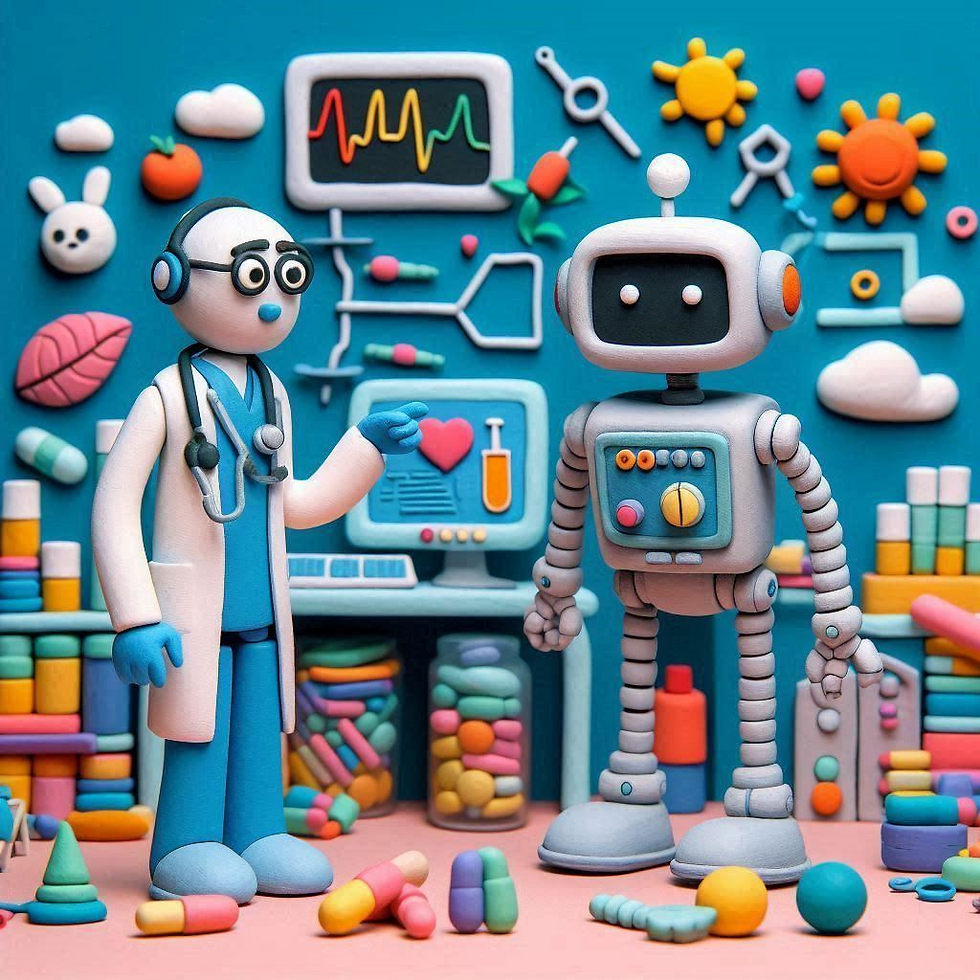
NLP and Machine Learning
Taking the same NLP technology, but used in a different way we have this study:
"AI-based approach to enable proactive identification of early lung cancer: a retrospective population health study and economic model" which was presented at the 2024 ASCO conference.
This research focused on using AI to improve the early detection of lung cancer by analyzing large datasets from electronic health records (EHRs). The study incorporated natural language processing (NLP) and machine learning (ML) to identify patterns that could indicate early-stage lung cancer. Specifically in a population with high socioeconomic disadvantages.
The benefit of such a model suggested by the study is a potential "left shift," meaning more cases being diagnosed at earlier stages (I or II rather than III or IV).
This is a massive deal with lung cancer. If we catch it early enough, it's curative!
A medley of AI in action, but how accurate is the model?
The detection potential of the model achieved an area under the receiver operating characteristic curve (AUROC) of 0.75, so what does that mean?
It suggests that the AI-based predictive model has a good, but not perfect, ability to distinguish between patients who have early-stage lung cancer and those who do not.
As you might have guessed, it means that in 75% of cases, it predicts correctly an early-stage lung cancer.
Another paper that caught my attention: Assessing eligibility for lung cancer screening using parsimonious ensemble machine learning models: A development and validation study. published in PLOS
They've whittled down the criteria required to predict your chance of lung cancer to just 3 variables, age, smoking duration, and smoking pack-years...
The area under the receiver operating characteristic curve (AUROC) for this model was 0.831, which is pretty impressive given that other prediction tools that are in this realm of accuracy use 11 variables.
Deep Learning Notable finds
At the risk of extending this blog post indefinitely, a few notable finds on the deep learning side of things that are already broaching future cancer prediction:
DeepSurv: A deep learning-based survival model that predicts individual cancer patient outcomes by learning from historical data. It adapts the Cox proportional hazards model with neural networks, offering personalized predictions for different types of cancers.
DeepRisk: A deep learning model designed to predict the risk of cancer recurrence by analyzing medical imaging data alongside clinical data. It has shown promise in breast cancer and prostate cancer risk predictions.
PathAI: An AI platform that analyzes pathology images to predict cancer diagnoses and outcomes. PathAI's models are being used to improve the accuracy of cancer diagnoses, particularly in breast and prostate cancers.
OncoAI: AI platforms that predict cancer risks by identifying novel biomarkers from vast datasets. These models can discover patterns in genomic, proteomic, or metabolic data that are linked to cancer risk, offering new avenues for early detection and targeted therapies.
So what next?
The future is bright. Some of the areas we are exploring now will be pushing the envelope forward in the future. Including Pancancer AI Models, that integrate data from multiple 'omics' sources (genomics, transcriptomics, proteomics, etc.) to predict cancer types, progression, and treatment responses. Which really is next-level stuff.
Another extremely popular area is Radiomics-based AI Models, which extract quantitative features from medical imaging data (e.g., CT, MRI) and use machine learning to predict cancer outcomes. And of course, circling back around to explainable cancer.
Those models that prioritise transparency, ensuring that AI predictions for cancer risk can be interpreted by clinicians. A crucial component for gaining trust and facilitating the integration of AI into clinical practice.
Many of the models I've found are at the forefront of research in transforming cancer prediction. Offering improvements in accuracy, personalisation, and the ability to handle complex, high-dimensional data that traditional models would struggle with.
Although many are still in the research phase or undergoing validation, they hold promise for widespread clinical adoption in the near future.

Final Thoughts
Watch this space! I think a personalised cancer prediction tool based on our very own bodies' makeup is on the way.... but it might be a while off, and it may be even longer before we can explain it!
See you next time!

[1] Grootes I, Wishart GC, Pharoah PDP. An updated PREDICT breast cancer prognostic model including the benefits and harms of radiotherapy. npj Breast Cancer. 2024;10(6). Available from: https://www.nature.com/articles/s41523-024-00612-y
[2] Predict Breast. Technical Information. Predict Breast Cancer Survival Prediction Tool. Available from: https://breast.predict.cam/about/technical/technical
Your blog was very much informational and is a good read . But when it is about healthcare GD Goenka healthcare academy is the one solution for top paramedical courses in Bhilwara Rajasthan in India.