How AI and machine learning can transform clinical trials data
We are living in an era of AI and machine learning, where these technologies are applied to various aspects of medicine and healthcare. However, one area that seems to lag behind is the format of clinical trials data.
What if we could use AI and machine learning to enhance the way we collect and present data in clinical trials?
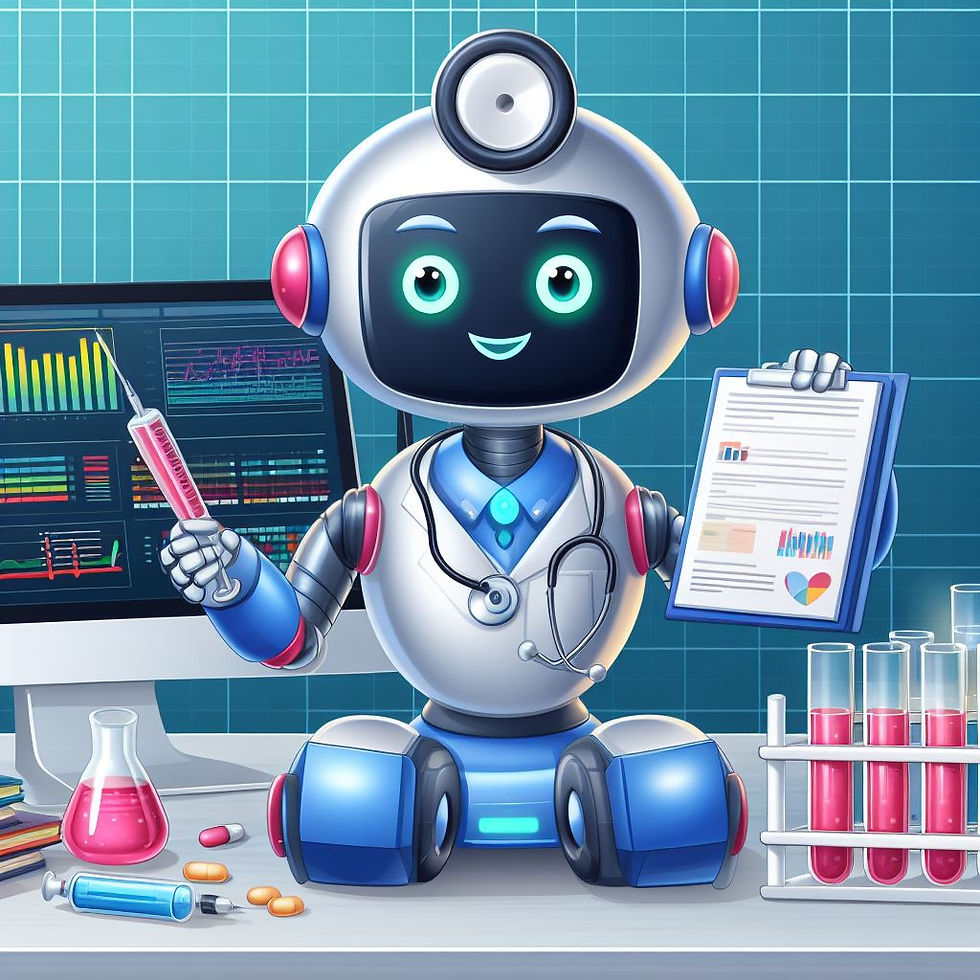
Imagine a trial data that is rich, deep, and layered.
Every point on our digital Kaplan-Meier chart is a patient. Not just a symbol or a statistic, but a person with all the details we usually gather when they enrol in a clinical trial.
Every point contains the demographics, sex, cancer type, subtype, medical history, current medications, etc. All of that data, in a single point, plotted. Now zoom out from that, and instead of seeing a 2D graph of patient survival percentage over time, we have a multi-dimensional matrix of data that can be analysed by AI. What if, when a patient visits a clinic, instead of giving them a rough estimate of their disease-free survival based on the trial evidence for a general population, we give them a personalized clinical trial outcome.
What if we can match them to the exact characteristics, pathology, medications, location of the metastasis, even the postcode they live in. AI makes this kind of data analysis possible.
Let’s take a concrete example: A high-risk renal cancer patient considering adjuvant Pembroluzimab after surgery. We have a clinical trial to support the use of it in this situation: https://www.nejm.org/doi/full/10.1056/nejmoa2106391 Here’s the paper, simple enough, just look at the Kaplan-Meier 24-month disease-free survival curve: 77% with Pembroluzimab, 68% with placebo. Seems like an easy choice, but… does this paper reflect your patient?
What if the patient in front of you was one of the <6% that had their metastatic disease removed during surgery.
Can you say this trial data is relevant for your patient? Maybe not.
But if we had the software to do it, we could capture that patient’s information before the clinic visit, apply it to the study and draw a realistic representation for them, of how people in their exact situation did.
Now imagine we could combine these results across multiple trials that captured data in the same format. Yes, we would need to think about the statistics, how studies are designed, powered and conducted to answer specific questions would need careful consideration, but what if we start using AI to look for the patterns we can’t.
Maybe finding connections between, say those on a statin and survival outcomes on pembrolizumab. The possibilities are endless.
The only way we can think about how to use our new technology is to step away from how we have always done it. New paradigms, new thinking and new methods will be how we solve our healthcare problems. Not just by taking the new technology and making it fit the systems we’ve always used, albeit in a more efficient or better way.
A mindset shift is needed.
Personalized medicine is here, and one way to make use of it is to make our data fit the patient in front of us. To me this seems like something we should be building. What do you think?
And if anyone is interested in helping me build this, please get in touch! :)
And so begins the first post for Machine Learning Monday. Although this is more of an idea dump than an explanation of how one might create such a thing, the next post in Machine Learning Monday we will definitely start to build a basic natural language processing system. However, truth be told my clinic ran over today and I had a huge clinic to prep for in the morning, so I had to keep this one short and sweet. :D
Robot trial HAL likes the idea as you can see below.
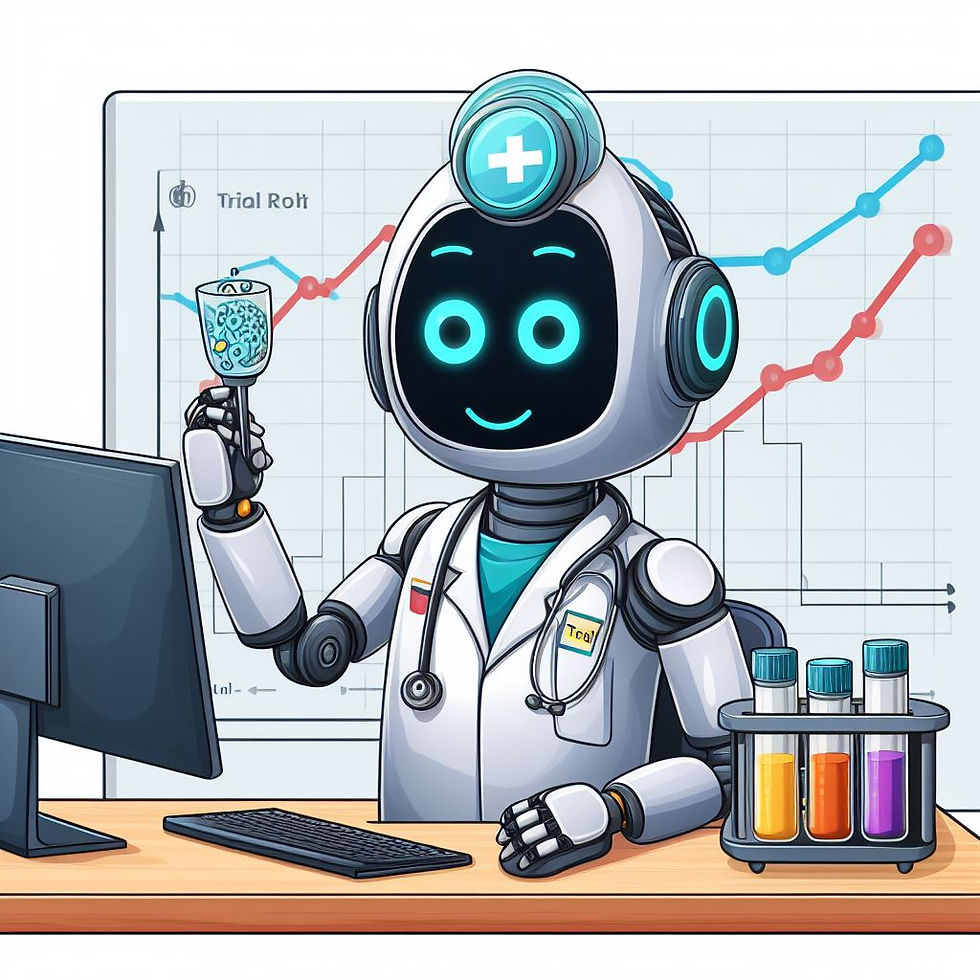
See you next time :D
Comments